Three Caveats of Electoral Forecasting
Important statistical context for FiveThirtyEight's model (or anyone's)
It’s only the end of the August, yet election season is in full swing. And with it has come the ubiquity of quantitative political forecasts. You can see model-projected odds for specific races and total Congressional seats cited in news stories, on social media, and even in campaign emails to donors.
As a data scientist who studied quantitative political science, it’s exciting to see this field gain popular traction. Yet as a statistical pedant with a professional history of asking annoying questions about what a model is really doing, there are nuances to interpreting such forecasts that aren’t always considered in conversations about their outputs. So here are three important factors to help contextualize these projections as you read them, as well as brief counterarguments to my own pedantry to explain why my nitpicks shouldn’t be seen as invalidating.
Note that I’m focusing mainly on Nate Silver and FiveThirtyEight’s model here because theirs is both the most-popular model and because they explain many of their modeling decisions in detail, but similar considerations and questions would be relevant for evaluating Race to the White House, Princeton Election Consortium, the Fair Model, or any other group that throws their hat into the midterm-forecasting ring.
Read on, and I predict you’ll be analyzing political forecasts like a pro!
Models expect what usually happens
As recently as a couple months ago, everything pointed to a likely Republican victory in November. You can start with the fact that the President’s party gaining seats in the House of Representatives in the midterm elections is roughly a once-in-a-generation event, and the Democrats’ current majority is so slim that any losses likely mean losing control of the chamber. Throw in Joe Biden’s deep unpopularity, widespread concerns about the economy and inflation, and the fact that the administration had been struggling to do much of substance despite its federal trifecta, and it looked like Democrats would soon be longing for the halcyon days when Joe Manchin was the biggest obstacle to enacting a progressive agenda.
The picture looks rosier now. Democrats have closed the gap in the generic Congressional ballot. They’ve had several stronger-than-expected performances in recent special elections, including Pat Ryan’s victory in the New York 19th District on Tuesday. Roe v. Wade being overturned was clearly a galvanizing event for the electorate. Combine that with gas prices coming down, passing the Inflation Reduction Act, and the particularly off-putting Republican candidates in this cycle, and there is reason for renewed optimism that the Democrats can not only maintain but expand upon their legislative majorities.
Yet FiveThirtyEight is still pessimistic — to the confusion of many people on social media. While they see Democrats as the favorites to hold the Senate, as of this writing they project the Republicans to win the House popular vote by a margin of between 2.0% and 3.8%, depending on the model version. This largely boils down to the fundamentals of the present political environment strongly favoring the GOP. If the recent polling trends hold, I assume the models will eventually be more bullish on the Democrats’ chances. But for the moment it’s hard to sell the algorithms on a Blue Wave supplanting the expected Red Tide.
Counterpoint: Models should expect what usually happens
Over the last 75 years, the President’s party has lost seats in all but two of the 19 midterm cycles. To vastly oversimplify the inner workings of a forecasting model, you could translate this into a Bayesian prior, giving the Democrats a baseline 11% chance to hold the House in 2023 before factoring in anything else. The polls and small-scale trends are important of course, but as tautological as it sounds, any empirical model worth its salt has to start with this iron law of U.S. politics.
This isn’t to say that FiveThirtyEight’s model (or any other) is weighing its myriad input variables correctly; maybe their skepticism at this stage is too strong. But there is no perfect way to pre-quantify an unprecedented electoral factor — like how the backlash to an extremist court reversing decades-old protections for privacy and health care will affect turnout. Short of fudging the model with an ad-hoc guesstimate, the intellectually honest approach is to base your projections on the data you are confident in, and acknowledge what you might be missing. Even if doing so makes your model slower to react to changing circumstances.
Models are feedback loops
There is a truism in statistics that the mere act of measuring something changes the way people behave. Picture driving on the highway and glancing at your speedometer. If you see that what had been your comfortable driving speed is notably above (or even below) the limit, you might reflexively tap on the brake (or accelerator), thus changing what the speedometer says. To use a political example, consider how voters are more motivated to turn out when polls show a close election than when the outcome is a foregone conclusion, and how lopsided turnout can impact the final results.
Publishing a formal election model creates extra opportunities for such feedback loops. Projecting a candidate to be a frontrunner enhances their credibility and gravitas in the minds of voters. Giving an underdog better-than-expected odds can lead to more media coverage and/or scrutiny. Identifying the closest races helps partisans guide fundraising efforts, which means the donation inputs that are supposed to be independent variables become reactions to the model’s outputs. It’s even reasonable to expect that the early projections even affect what surveys are available to feed into the it later. “There’s a lot of selection bias in which races are polled,” FiveThirtyEight’s model-explainer reads. “A House district usually gets surveyed only if one of the campaigns or a media organization has reason to think the race is close.”
The clearest example of this effect comes in FiveThirtyEight’s “Deluxe” model, which is shown by default when exploring their forecasts. The Deluxe version’s inputs include qualitative projections from outside pundits, which are useful datapoints with real predictive power. But they are also surely influenced by the existing model projections! Even if the politicos in question don’t have FiveThirtyEight open in another tab while they update their ratings, pundits who specialize in analyzing electoral trends must be aware of what the most-popular forecasting model says. And even if they somehow aren’t, the fact that the prognosticators are looking at the same data as the model creates potential for multicollinearity, the condition of convolutedly spreading a single factor across two different variables.
Counterpoint: Adding these inputs improves the accuracy
FiveThirtyEight’s methodology page explains that my theoretical concerns are misguided. The Deluxe model with pundit ratings reduces the number of miscalled Congressional races in training data by 18% compared to the polls-only “Lite” version, and by 6% relative to the polls-and-other-quantitative-factors “Classic” iteration. At the end of the day, accuracy matters much more than some internet writer’s pedantry. Having said that, I would be interested to know if those results held in out-of-sample testing — i.e., data that the models didn’t have access to while they were being built — and whether the Deluxe version ends up retaining its advantage now that the potential feedback loop is in place.
Model evaluations focus on Election Day
FiveThirtyEight has rightly received — and taken — a lot of credit for their model’s relative bullishness about Donald Trump’s chances of winning the 2016 election. Their final forecast gave Trump a 29% chance of winning by the then-equivalent of the Lite model, and 28% by the predecessor of Classic. To bastardize the idea of a probability estimate by putting it in binary terms, this made them the least-wrong of the public forecasting systems, all of which had Hillary Clinton as the heavy favorite.
Yet each of FiveThirtyEight’s then-two models didn’t just record one projection: they made 154, one for each day from June 8 through November 8 (not including instances in which they were updated multiple times in a day). And the most-impressive showing for the site’s forecasts came on July 30, when the Lite model had Trump as the favorite. It was the slimmest of margins — 50.1% chance of winning for Trump, compared to 49.9% for Clinton — yet to my knowledge it was the only time when any serious quantitative model favored Trump prior to Election Day. (By the same token, touting their relatively conservative 72% final odds for Clinton obscures the fact that she surged to be an 89% favorite merely two weeks after reaching her nadir.)
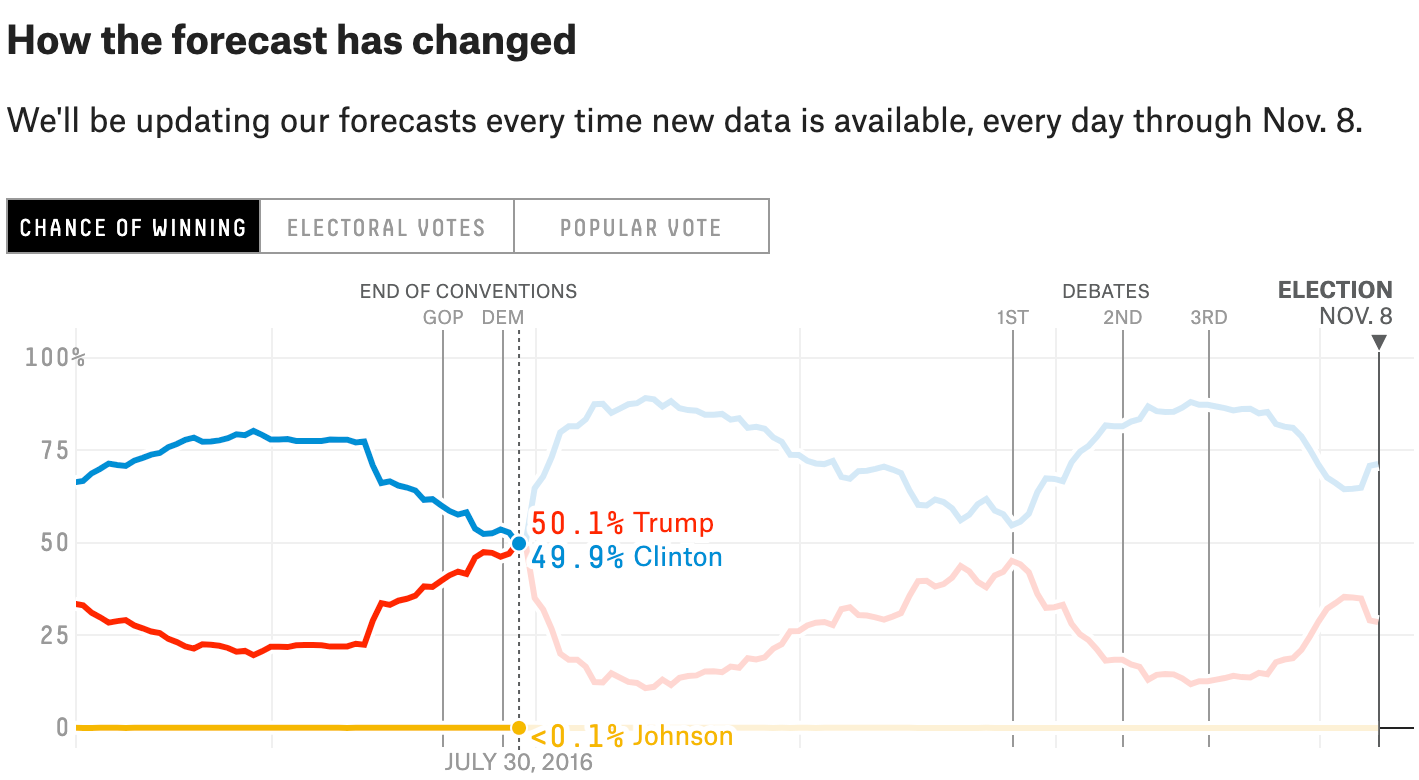
Why isn’t the fact that their model correctly predicted the winner at least once when no one else’s did a major bragging point for FiveThirtyEight? It’s especially surprising since they are admirably transparent about evaluating their forecasts over time in the aggregate. As far as I can tell, it’s because Silver (along with most people who track such things) considers the final forecast to be the main one by which a system should be judged.
While the projections are valid and probably well-calibrated even several months before the election, the intervening ebbs and flows of the forecast before its terminus won’t be (publicly) rehashed the way the final numbers will. Keep that in mind as you consider how big a grain of salt with which you should take the forecasts now.
Counterpoint: The final forecast is more important
To dramatically oversimplify how electoral modeling works, there are two broad goals of a forecasting system: predicting what the political environment will be like on Election Day, and translating the state of the country into voter behavior. (For those who have been following FiveThirtyEight for a while, consider the difference between the since-deprecated “Now-cast” and the conventional models.) The latter question is frankly more interesting, but the former is hugely important and very difficult, too…at least until Election Day. Thus a model’s final forecast is both a more-straightforward exercise in political science and has a lower theoretical margin of error than at any other point in time.
This logic makes sense to me; if I had an election-forecasting system, I would probably care most about its final accuracy, too. But when every update before votes are cast is presented as a serious forecast, a rigorous review of a model’s accuracy or comparison to other ones should take every prediction into account.